Historically, refinery operators have performed regular inspections to identify corrosion damage and determine if equipment must be replaced. These inspections are costly, therefore, processes, such as risk-based inspection methodologies to prioritize inspections were established.
The use of a detailed, rational process, which is derived from risk and potential equipment damage results, aids in prioritizing inspections. Probability and consequence are the key components of risk assessment. Data regarding damage mechanisms and their effect on the life of the equipment, along with criticality of failure are typically considered.
The common assumption that corrosion rates remain constant and that asset integrity degrades at a constant rate is often incorrect. Inspections reveal locations in which corrosion rates were higher than anticipated, but cannot show when the damage took place, nor how to mitigate it or avoid potential damage in the future. Additionally, inspection cannot divulge whether particular processes of metallurgical parameters are the cause of damage. Table 1 illustrates historical methods vs. software-prediction methods.
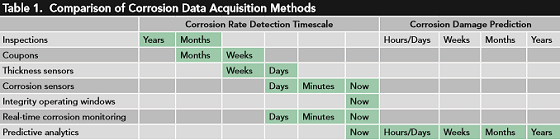
Challenges of Refinery Corrosion
Because refinery operations are so complex, and obtaining accurate asset integrity information has proven to be a challenge, a need exists for quantifying corrosion. Corrosion must be characterized and managed amongst different critical process units and parameters. These requirements must be met to obtain an accurate picture of asset integrity, without which destructive and expensive corrosion failures may occur.
Challenges exist in managing and understanding corrosion in refineries. Processes and operations often involve intricate acts of engineering, including changing process feed and chemistry, combined with the need to meet demanding production targets and the challenges grow exponentially. One of the chief obstacles is the absence of quantified corrosion data and the correlation with operating and process conditions.
The most effective corrosion management system involves multiple approaches, rather than through instrument and coupon-measured corrosion rates alone—and historically an inadequate amount of data exists. With that in mind, researchers Sridhar Srinivasan, Pierre Constantineau, and Kwei Meng Yap1 of Honeywell Process Solutions, along with support from leading global refinery operating companies, developed comprehensive engineering data and data-driven prediction models.
This novel software framework addresses the most common refinery corrosion issues, such as sour water corrosion, naphthenic acid corrosion, crude distillation unit (CDU) overhead corrosion, amine corrosion, and sulfuric acid (H2SO4) alkylation. The researchers incorporated intelligent predictive analytics paired with process historian to provide corrosion damage information in real time.
Experts working within the Joint Industry Projects (JIP) initiative utilized engineering data, advanced phase behavior, and numerical modeling to develop multiple models that support accurate prediction and quantification of corrosion. They have developed models for: sour water corrosion in refinery units, high-temperature naphthenic acid and sulfidation attack in crude distillation and vacuum distillation units, salt under deposit and aqueous corrosion in CDU overhead systems, corrosion in amine treatment units, and corrosion in sulfuric acid alkylation units.
Utilizing a Proven Methodology
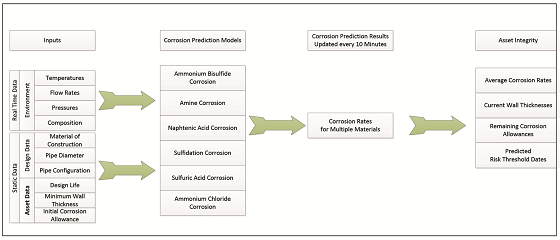
The researchers emphasize the importance of utilizing a proven methodology that produces its own corrosion data to create reliable predictive corrosion models. This helps to eradicate the problems with process plant data, such as:
• Using measured corrosion rates for one operating point, not the average of multiple operating conditions for one corrosion rate measurement
• Controlling process conditions tightly around their operating point
• Accurately measuring corrosion rates for a wide range of materials
• Using a number of operating points that far surpasses the range of normal operating conditions
• Accurately modeling flow conditions through appropriate characterization of vapor liquid equilibria and multiphase flow
• Supplementing wild-card conditions to the experimental programs to validate the model, pinpoint any hidden variables, and study the ways specific damage mechanisms operate
• Tuning and validating models by evaluation with actual refinery measurements
Accurate corrosion models must be developed using the fundamentals of corrosion, thermodynamics, and chemical engineering principles. The ability to correlate operating conditions to corrosion rates allow for robust prediction models, enabling refineries to end processing their own operating data and attempting to infer useful statistical models. Instead, they can use reliable predictive models that convert real-time operating process data into real-time corrosion rates. Figure 1 shows that temperature, pressure, and flow rates—the most important variables in corrosion prediction—are measured and updated in real time.
Corrosion Prediction Framework
The researchers describe their prediction framework, called Predict†-RT2, as integrating “intelligent data analytics, extensive data modeling, and process correlations to provide proactive corrosion management through online, real-time corrosion prediction and monitoring.”1 This real-time technique facilitates operators to see corrosion as an operating process variable. Dynamically updated corrosion-prediction rates transpire as operational conditions change. Figure 2 illustrates the corrosion prediction framework.

A functionality was incorporated into the software that combines accurate corrosion rate data sets and state-of-the-art predictive analytics models. This precision is imperative to accuracy in such a complex, difficult task. The true value is attained once this information is made available in real time.
This novel software framework that integrates vast research and experimental data with intelligent predictive analytics provides refinery operators with real-time information. Doing so allows operators to achieve operational excellence, maximize asset performance while minimizing costs, and maintain high safety and environmental standards.
† Trade name.
Reference
1 S. Srinivasan, P. Constantineau, K.M. Yap, “Improved Mechanical Integrity through Real-Time Process Monitoring and Predictive Analytics,” CORROSION 2019, paper no. 13286 (Houston, TX: NACE International, 2019).